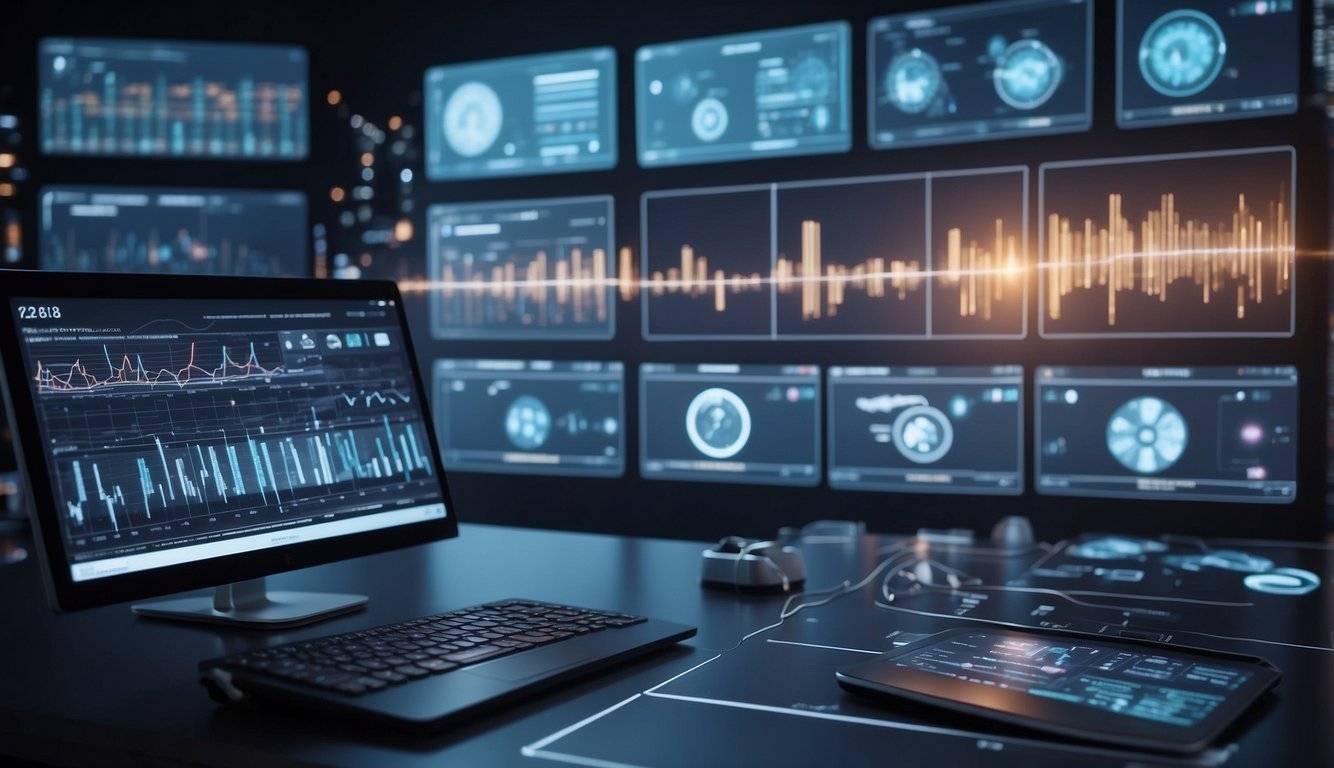
As someone who works as a researcher in a hospital setting, I have heard a lot about analytics. In the beginning, it sounded to me like yet another hip industry buzzword that was supposed to raise our rankings against other hospitals to get more patients in the door.
As time progressed, I realized how important analytics can be to the improvement of medical care. “Analytics,” or analyzing large-scale patient data, is a way of understanding trends in disease and treatment outcomes to improve healthcare. As computer technology has improved, more and more patient data can be stored, retrieved, and even analyzed by statisticians and scientists to find trends and create innovative solutions to diseases from COVID-19 to juvenal diabetes.
In this article, we will discuss how predictive AI Analytics for healthcare is beginning to become useful in healthcare analytics to improve patient care.
Table of Contents
Predictive AI analytics in healthcare is like having a crystal ball, but instead of magic, it’s powered by data and algorithms. It involves crunching tons of health-related information to forecast future health events or conditions. Healthcare professionals can use these insights to make better decisions, tailor treatments, and even nip potential health issues in the bud before they become serious.
How does AI fit into this equation? Artificial intelligence (AI) learns from historical data—imagine it browsing through countless electronic health records to detect patterns everyone else might miss. It’s not just about the numbers, though. With machine learning, AI gets smarter over time, tweaking its algorithms to improve its predictions, whether it’s flagging high-risk patients, estimating hospital resource needs, or predicting disease trajectories.
Looking to the future, this fusion of healthcare and technology is not slowing down. As predictive analytics gets more woven into daily healthcare practices, it’s set to transform how doctors and hospitals work. They’ll be proactive, not reactive—catching health issues early and managing diseases more effectively. Plus, this tech can help with the business side of things, shaping up resource allocation and operational efficiency.
Here’s a snapshot of where predictive AI analytics can be a game-changer in healthcare:
- Anticipating Patient Risks: Identifying patients who might be at risk of adverse events.
- Resource Optimization: Allocating hospital resources like staff and beds more efficiently.
- Disease Management: Predicting disease progression to customize treatments.
- Operational Efficiency: Streamlining hospital operations, reducing wait times and costs.
Click the video below to see a clip from syncbricks about Google AI and how AI could improve global access to healthcare.
Implementing Predictive Analytics in Clinical Settings
Enhancing Patient Care with AI
AI improves patient care by predicting medical conditions and assisting in clinical decision-making. For example, machine learning can anticipate the risk of diabetes or stroke, enabling early interventions. This elevates the quality of care patients receive.
Operational Advantages of Predictive Models
Using predictive models, healthcare facilities can allocate resources more effectively. Accurate forecasts of patient influx and the likely demand for different services will improve operational efficiency and reduce waste.
AI in ICU: Managing Critical Care
In the ICU, AI-driven predictive models help manage critical care by monitoring patient outcomes. These systems detect subtle changes in patient status that might signal a deterioration, guiding immediate attention to those who need it most.
Improving Diagnosis and Treatment
Radiology benefits from AI by improving diagnosis accuracy. Algorithms analyze images faster and sometimes more accurately than humans, which could lead to better treatment plans for diseases like cancer.
Utilizing Big Data and EHRs
Big data and electronic health records (EHRs) feed AI systems with the historical data needed for accurate predictions. Leveraging this data, predictive analytics can inform clinical decision-making, enhancing patient safety and care.
Predictive AI and Chronic Diseases Management
AI models monitor disease progression and comorbidities in patients with chronic diseases, offering insights for better management. This proactive approach can improve patients’ quality of life and reduce complications.
Reducing Readmissions with Predictive Insights
Predictive analytics aim to reduce hospital readmissions by identifying patients at risk. This not only ensures patient safety but also decreases unnecessary lengths of stay, resulting in cost savings for both patients and healthcare providers.
Predictive AI Applications in Healthcare
Case Studies: Predictive AI Impact on Outcomes
Predictive AI has shown significant improvements in patient outcomes across various cases. For instance, a study indicated that AI helps in accurately predicting patient deterioration and readmissions. In one clinical study, predictive AI models identified patients at risk of sepsis, thereby enabling timely interventions.
Addressing Pandemics with Predictive AI
During the COVID-19 pandemic, predictive AI played a pivotal role in forecasting outbreak patterns, which helped healthcare providers prepare and manage resources. AI models provided visibility into the virus’s spread and predicted hotspots, aiding pandemics responses.
Social Determinants and Patient Behavior
AI takes into account social determinants of health and patient behavior to predict outcomes. With this data, healthcare organizations can identify at-risk individuals and provide preventive care, cutting down on severe cases and hospital readmissions. The influence of lifestyle choices on health is also easier to analyze with AI.
Innovations in Medical Imaging
In medical imaging, AI-driven tools, especially using deep learning, are enhancing radiology by boosting the accuracy of diagnosis. AI models can detect abnormalities in imaging data that are sometimes missed by the human eye, leading to earlier and more precise interventions.
AI-Powered Genomics and Personalized Care
The field of genomics is being transformed by AI, paving the way for precision medicine. AI analyzes genetic information to aid in the development of personalized care plans, which can dramatically alter patient outcomes. Advances in this area suggest a promising future for individualized health strategies and treatments.
Technological Framework and Data Handling
Integrating AI with Internet of Things (IoT)
Pairing AI with the Internet of Things is a game-changer, especially with wearable devices. They’re constantly gathering health metrics in real-time which AI systems analyze to alert healthcare providers about potential health issues before they worsen.
Deep Learning and NLP in Healthcare
Deep learning crunches complex datasets, while Natural Language Processing (NLP) interprets free-text notes in electronic health records. This powerful combo enables clinical decision support systems to provide more nuanced and effective patient care.
Enhancing Data Mining and Analytics
The use of advanced data mining techniques and analytics is critical. Such machine learning algorithms can identify patterns pointing to disease risks, leading to earlier interventions and better quality healthcare services.
Security and Privacy in AI-Enabled Healthcare
Protecting patient data in the AI space is paramount. Ensuring security and privacy involves encrypting data transfers and adhering to privacy regulations, building patient trust and safety in technology-driven medical care.
Improving Predictive Models with Analytics
Predictive models in healthcare benefit from advanced analytics. They use historical and real-time data to make predictions, with calibration fine-tuning their accuracy. This results in sharper clinical insights and patient-centered care strategies.
Dealing with Missing Data in Medical Analytics
Handling missing data in medical datasets is tricky but essential. Imputation strategies and advanced algorithms can help maintain the integrity of predictive outcomes even when data points are absent.
Leveraging Social Media for Health Insights
Social media streams are treasure troves of behavioral insights. With the right analytics tools, healthcare professionals can tap into this data, identifying public health trends and patient behavior in a non-intrusive, cost-effective way.
Click the video below to see Eye on Tech explain predictive modeling.
Challenges and Future Directions
Confronting AI Implementation Barriers
Healthcare organizations often grapple with technical challenges when implementing AI technologies. Compatibility with existing systems and ensuring patient safety are amongst the predominant hurdles. They must ensure that predictive analytics tools are seamlessly integrated into clinical workflows to support, not hinder, healthcare professionals.
AI in Clinical Decision Support Systems
Incorporating AI into clinical decision support systems is an evolving frontier. The goal is to provide substantial support in decision making, enhancing the accuracy of clinical predictions. The effectiveness of these systems hinges on the quality of the predictive model and its integration with patient data.
Optimizing Staffing and Resource Management
Predictive AI’s application in staffing and resource management serves to dramatically improve operational efficiency. It allows for a more dynamic allocation of resources, potentially reducing wait times and increasing patient care quality while maintaining financial sustainability for health systems.
Predictive AI in Academic and Community Hospitals
Academic and community hospitals are utilizing predictive analytics to tailor clinical care to individual patients. Different health systems adopt various AI strategies, impacting staffing, patient outcomes, and educational purposes.
Advancements in Disease Recognition and Management
Disease recognition is a domain where machine learning shows promise. The future could see AI early identifying conditions from imaging scans, leading to quicker medical treatment and potentially better patient outcomes, especially in fast-progressing diseases.
AI’s Role in Cardiac and Renal Disease Care
AI’s predictive capabilities are applied to cardiac problems and renal disease, aiming to forecast patient deterioration. These tools can identify subtle changes in patient data, prompting early intervention and possibly decreasing mortality rates.
Tackling Sepsis and Other Acute Conditions
Sepsis poses challenges due to its complex nature. Predictive AI analytics support the prompt identification and treatment of sepsis, aiding in minimizing patient care delays and improving survival rates.
Monitoring Patient Health with Predictive Devices
Wearable devices equipped with predictive AI algorithms are augmenting patient monitoring outside traditional clinical settings. They hold the promise of advancing patient safety by detecting early signs of patient deterioration, all while allowing patients to maintain their normal daily activities.

Frequently Asked Questions
What tools are commonly used for implementing predictive analytics in healthcare?
Tools like electronic health records (EHRs), data from wearable devices, and machine learning platforms are integral for predictive analytics in healthcare. These facilitate the analysis of vast datasets to predict patient outcomes.
Can you cite some examples of how AI algorithms are being applied in the medical field?
AI algorithms help in early disease detection, such as identifying potential cases of breast cancer through mammogram analysis. They also assist in predicting patient readmissions and tailoring individual treatment plans.
Could you name a few companies that specialize in healthcare predictive analytics?
Companies like Health Catalyst and IBM Watson Health are prominent in the healthcare predictive analytics space. They offer solutions that forecast clinical, operational, and financial needs within healthcare organizations.
How has predictive analytics impacted actual healthcare case studies?
Case studies often highlight the use of predictive analytics in reducing hospital readmissions and managing chronic diseases by anticipating flare-ups and modifying treatment proactively.
What advancements might we expect in the area of predictive analytics for healthcare?
As technology evolves, advancements may include more accurate genome sequencing analytics and real-time predictive insights that could lead to faster diagnoses and more effective interventions.
What practical applications does predictive analytics have for ongoing healthcare projects?
In ongoing projects, predictive analytics is being applied to optimize resource allocation, enhance patient care pathways, and identify patients at risk of adverse events, thereby improving the efficiency and effectiveness of healthcare delivery.
2 thoughts on “Predictive AI Analytics for Healthcare”
I have read some excellent stuff here Definitely value bookmarking for revisiting I wonder how much effort you put to make the sort of excellent informative website
Attractive section of content I just stumbled upon your blog and in accession capital to assert that I get actually enjoyed account your blog posts Anyway I will be subscribing to your augment and even I achievement you access consistently fast