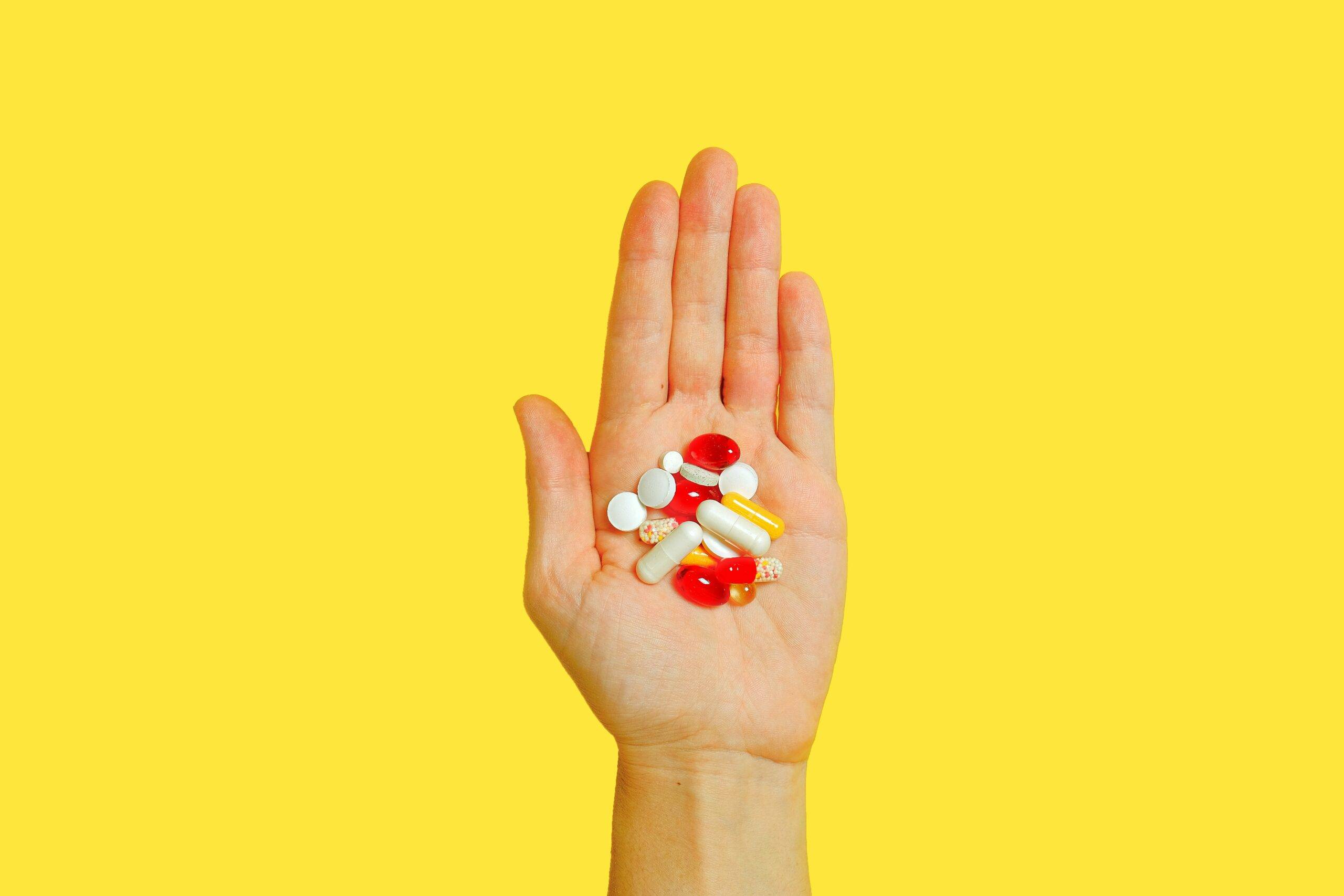
Wouldn’t it be great if we had a drug for everything? Imagine if no matter what health problem you were struggling with, you could go to your primary, ask for a prescription, and abracadabra, you were cured. It has been the dream of medical science since the dawn of time to be able to simply cure everything and anything that could possibly give you the sniffles. Or heart disease. Or obesity. Or cancer.
While huge discoveries have been made even in the past five years, the list of cures that we have falls far short of this fantasy of cures for all diseases. Recently, the internet has been a buzz with excitement over a new technology called artificial intelligence (AI). Could it be that this new computer based technology could hold some of the answers to diseases that have so far escaped us? It turns out that this idea is not totally unfounded as many look to this new technology to give us new insight into how to find the next big lifesaving drug. In this article, we will explore how one type of AI, called deep learning, might be the next big thing to move the drug discovery field forward.
Table of Contents
Understanding Deep Learning in Drug Discovery
The field of artificial intelligence (AI) has made significant strides in recent years, particularly in a subdomain known as deep learning. This advanced technology is garnering attention for its potential in drug discovery, as it helps to process complex molecular data and predict the effectiveness of potential therapeutic compounds.
Deep learning, which falls under the umbrella of machine learning, involves the use of artificial neural networks to model and analyze data. These networks can recognize patterns and learn features from vast amounts of information, enabling them to make accurate predictions and provide insights for various applications, such as image and speech recognition.
In the context of drug discovery, deep learning models can assist scientists in identifying chemical structures that interact with specific biological targets. This allows researchers to prioritize potential drug candidates based on their ability to impact the underlying molecular mechanisms of a disease, ultimately expediting the process of developing effective treatments.
Deep learning techniques have the potential to revolutionize the ways in which researchers approach drug development by offering new approaches for understanding complex biological systems. For instance, deep learning models can process large-scale genomic data and predict how genetic variations may impact a drug’s effectiveness, or identify potential targets for drug intervention in patients with specific genetic profiles.
Essential Concepts in Deep Learning
Deep learning is a subfield of artificial intelligence that focuses on training complex and powerful models, such as neural networks, enabling machines to learn hierarchies of patterns and representations from raw data.
One popular type of neural network is the convolutional neural network (CNN), which is primarily used for image analysis and computer vision tasks. CNNs use convolutional layers to scan input images for local patterns and pooling layers to condense information, making them more robust to variations in position and scale.
Artificial neural networks (ANNs) are the general term for any deep learning model that uses interconnected layers of simple processing units, known as neurons. ANNs are designed to learn and recognize complex patterns by adjusting the weights of connections between neurons during training. This process enables ANNs to automatically extract useful features from raw data and perform tasks like classification and regression.
Another essential concept in deep learning is the recurrent neural network (RNN), which is specifically designed for sequence data such as time series or natural language text. RNNs handle sequential data by maintaining internal states that can be updated as new data points are processed, allowing them to remember information from past inputs and predict future values, making them well-suited for tasks such as speech recognition, language modeling, and translation.
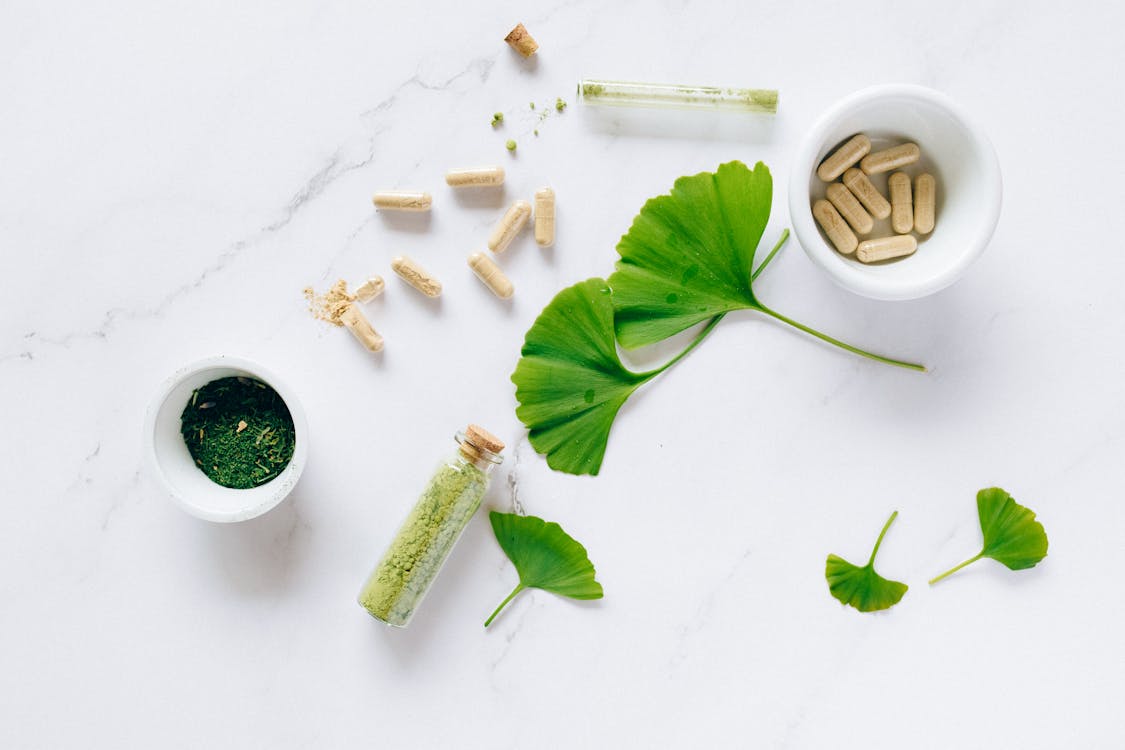
Drug Discovery and Designing
Deep learning has significantly impacted the field of drug discovery and design. It has paved the way for new and innovative techniques, such as de novo drug design and cheminformatics, which help in optimizing the drug discovery process. The integration of AI and deep learning models not only accelerates the research process but also reduces the risk and expenditure in clinical trials.
De novo drug design focuses on designing new molecules from scratch using computational techniques. In this approach, deep learning models generate novel chemical structures that can potentially interact with specific drug targets. Cheminformatics, on the other hand, combines the power of computer science and chemistry to analyze and predict the chemical and biological properties of molecules. These techniques enable faster identification of drug candidates, ultimately improving the efficiency of the drug discovery process.
Several deep learning tools are available for the public domain to aid in drug discovery and development. For instance, DeepCPI, DeepDTA, WideDTA, PADME DeepAffinity, and DeepPocket are some of the well-known tools that focus on identifying drug targets and drug-target interactions. These tools utilize the power of deep learning algorithms to predict molecular structures, functions, and interactions that are crucial in the design of effective drugs.
Additionally, explainable artificial intelligence (XAI) provides insights into the decision-making process of deep learning models, ensuring a higher level of transparency and trustworthiness. This is particularly important in the drug discovery domain, where understanding the underlying factors that lead to a specific prediction is essential for validation and optimization.
Role of Bioinformatics and Big Data
Bioinformatics plays a crucial role in drug discovery by providing essential insights into the complex molecular world. It enables researchers to analyze multi-omics data from various sources, such as genomics, proteomics, and metabolomics. This vast amount of biological data, often referred to as “big data,” enables scientists to scrutinize intricate relationships between molecules, pathways, and diseases, helping to identify promising drug targets and candidates.
The use of artificial intelligence (AI) and machine learning in the field of bioinformatics has significantly advanced drug discovery efforts. By harnessing the power of AI, researchers can sift through large, complex datasets much more rapidly and accurately than traditional methods. This enables the identification of patterns or trends that could inform the design and optimization of potential therapeutic agents. Moreover, deep learning tools are proving invaluable for advancing drug discovery and development, providing improved accuracy and efficiency in identifying promising candidates.
One of the significant challenges in dealing with big data is the efficient mining and analysis of such vast and diverse datasets. Data mining techniques, such as clustering, classification, and regression, have been applied to bioinformatics studies to drive insights from the information. These methods enable researchers to identify patterns and make predictions, such as potential adverse effects of compounds, which can then be tested in experimental settings.
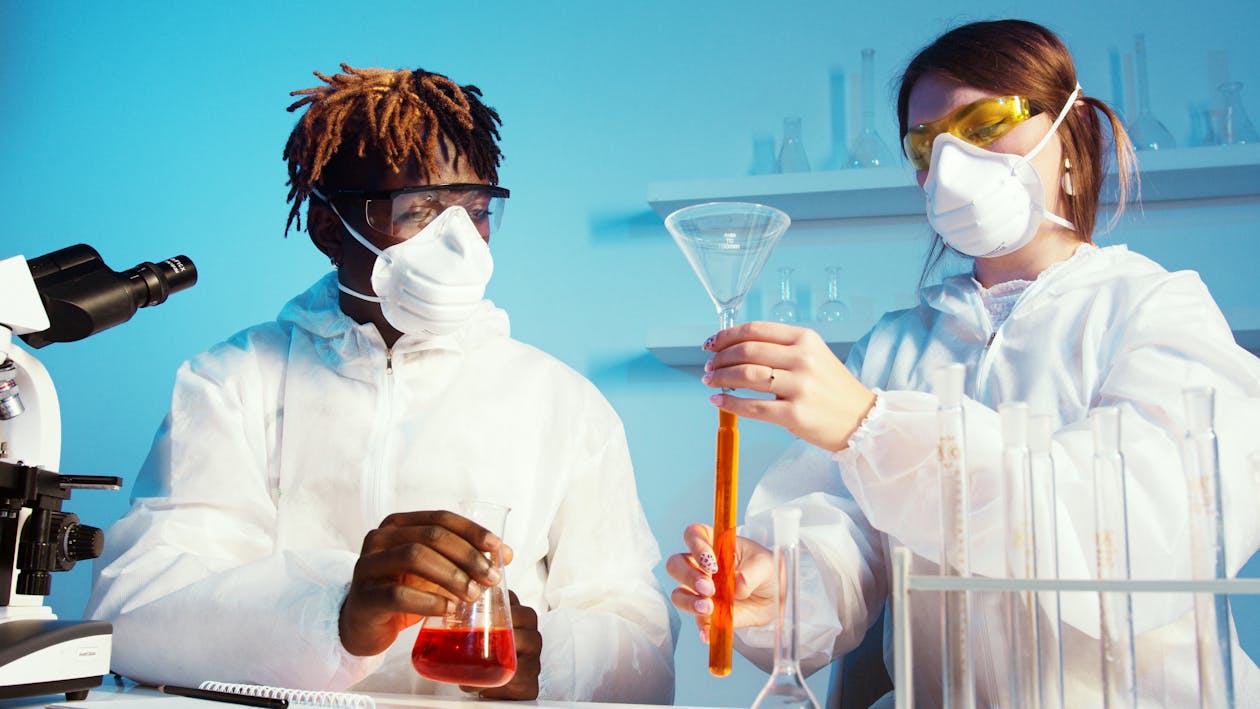
Computational Approaches in Drug Discovery
The field of drug discovery has significantly evolved over the years, and computational approaches have played a crucial role in advancing this domain. These innovative methods involve a range of techniques to streamline and optimize the drug development process, including virtual screening, computer-assisted drug discovery, and other computational tools.
Virtual screening is one essential computational approach, which aids in rapidly evaluating large molecule libraries for potential drug candidates. Through this method, researchers can identify which molecules have the desired properties such as binding affinity, and minimize experimental efforts. Virtual screening provides a cost-effective and efficient way to select the best drug candidates before investing time and resources in the later stages of drug development.
Another vital aspect of computational approaches is computer-assisted drug discovery, which involves combining experimental and computational techniques to accelerate the process of identifying, optimizing, and evaluating new drug candidates. This can be achieved through a variety of methods, such as molecular docking, molecular dynamics simulations, and machine learning algorithms. By integrating these tools, researchers can gain insights into the molecular and biological processes crucial for effective drug development.
Advancements in computational techniques have spurred the growth of various informatics tools, such as bioinformatics, pharmacoinformatics, and cheminformatics, which help speed up the drug development process. These tools have become indispensable in the field, as they merge critical data from various sources and provide researchers with comprehensive insights into potential drug-target interactions.
Machine Learning and Its Variants
Machine learning (ML) is a type of artificial intelligence (AI) that enables software applications to become more accurate in predicting outcomes. There are several ML approaches, including supervised learning, unsupervised learning, and reinforcement learning, each with its unique applications and advantages in drug discovery.
Supervised learning is a popular ML approach where an algorithm is trained on a dataset with known outcomes. This learning process allows the model to find relationships between data attributes and predicted outcomes. In the drug discovery field, supervised learning can help predict the effectiveness and possible side effects of new compounds by being trained on datasets containing known molecular properties and biological activities.
Unsupervised learning differs from supervised learning in that it deals with datasets without labeled outcomes. Instead, the algorithm identifies patterns, clusters, or relationships within the data. For drug discovery, unsupervised learning techniques can explore molecular data without prior knowledge, leading to the discovery of new relationships, such as novel molecular properties or potential applications.
Reinforcement learning is a unique ML approach where an agent learns to make decisions by interacting with an environment and receiving feedback in the form of rewards or penalties. In the context of drug discovery, reinforcement learning can adapt search strategies based on the ongoing experimental results, allowing for the optimization of drug properties through iterative design processes.
Prediction and Classification Techniques
Deep learning continues to make exciting advances in the field of drug discovery. It employs various prediction and classification techniques to streamline the process and enhance overall outcomes. Among these techniques, you’ll find methods such as classification, regression, and support vector machines. Each one plays a crucial role in improving the accuracy of drug predictions and the overall drug discovery process.
Classification is widely used in the realm of deep learning, enabling researchers to categorize data into specific groups. For instance, drug responses can be classified based on their effectiveness, helping experts to determine the best possible treatments for patients. Deep learning algorithms then assist in the classification process, as they excel in handling huge datasets and identifying patterns that humans might miss.
Next up is regression, a technique that focuses on predicting continuous values. In drug discovery, regression models play a vital role in predicting the relationship between various molecular properties and the effectiveness of potential drug candidates. Deep learning algorithms can enhance the accuracy of these predictions, quickening the pace of drug development and saving valuable time and resources.
Support vector machines (SVM) are another essential technique in drug discovery. As a type of machine learning, SVM is particularly effective in classification and regression tasks. It is adept at identifying patterns in complex data structures and offers top-notch prediction performance, even when faced with small sample sizes.
To achieve high prediction accuracy, researchers often combine various techniques and models from deep learning, utilizing the strengths of each method. This approach ensures optimal results in drug discovery, enabling the development of effective and targeted treatments.
Evaluating Efficacy and Side-Effects
Deep learning has shown great potential in drug discovery by improving the accuracy and speed of evaluating a drug’s efficacy while predicting side effects. The application of deep learning techniques in this field can play an essential role in understanding ADMET (Absorption, Distribution, Metabolism, Excretion, and Toxicity) properties, which are crucial in determining a drug’s success throughout its development journey.
In recent years, deep learning algorithms have been employed to assess drug-drug similarity interactions (DDIs), which help identify the relationships between different drugs and their possible combined effects. By analyzing DDI patterns, researchers can gain valuable insights into drug synergy or potential adverse effects when two or more drugs are taken together. An integrative review highlights the advancements deep learning has brought to this area.
Another vital aspect of drug development is predicting drug-side effects. Deep learning models can analyze large databases containing drug and patient information, aiding in the early detection of potential side effects. This allows scientists to refine the drug’s composition and dosage, resulting in a safer and more effective medication for patients.
Here are a few ways deep learning contributes to evaluating efficacy and side-effects:
- Analyzing drug-target interactions (DTIs) to predict potential drug effects and uncover new therapeutic applications.
- Improving drug sensitivity and responsiveness predictions, which facilitate a drug’s personalized administration.
- Enhancing our understanding of molecular mechanisms and the interaction networks that underlie drug action.
- Reducing the time and cost associated with drug development by accelerating the process of filtering potential drug candidates.
Protein Structure and Drug Target Interaction
In the field of drug discovery, deep learning plays a vital role in predicting protein structures and understanding drug-target interactions. This innovative approach has helped researchers develop new therapeutic compounds and advance the pharmacological landscape.
One essential aspect in drug discovery is the Quantitative Structure-Activity Relationship (QSAR) modeling. QSAR uses computer algorithms to predict the activity of potential drug candidates based on their chemical structure. Deep learning techniques have significantly improved the performance of these models, allowing for more accurate predictions and speeding up the drug development process.
Protein structure prediction is another crucial task that deep learning can aid tremendously. Accurate protein structure prediction is essential for understanding how a drug candidate interacts with its target. With the help of deep learning techniques, researchers are now able to predict protein structures with exceptional accuracy, opening up new possibilities for drug design.
Drug-target interaction prediction is vital for identifying potential therapeutic compounds and their corresponding protein targets. In recent years, deep learning methods have been developed to predict these interactions more effectively, such as DeepCPI and GraphDTI. These methods utilize advanced algorithms to analyze vast amounts of data, providing better insight into the complex relationship between drugs and their targets.
Additionally, deep learning has improved our understanding of drug–target interactions by modeling the mechanisms of action for various compounds. These models consider not only the binding affinity of a drug to a single protein but also the complex signal transduction cascades and molecular interactions that lead to specific therapeutic effects.
Role of Clinical Studies in Drug Discovery
Deep learning has revolutionized many aspects of drug discovery, particularly with its ability to streamline various stages of the pharmaceutical industry. One of the key areas that can benefit from deep learning advancements is clinical studies. Clinical studies, including preclinical research and clinical trials, play a vital role in determining the safety and efficacy of novel therapeutics before they are introduced into the market.
In the early stages of drug discovery, preclinical studies focus on understanding the drug’s mechanisms, its potential side effects, and optimal dosage. By employing deep learning models, researchers can quickly analyze massive amounts of data to identify promising drug candidates. Integrating these techniques into preclinical research has the potential to reduce the time and resources required for studying drug-target interactions and drug toxicity.
When it comes to clinical trials, a well-designed trial is crucial for validating the safety and efficacy of novel treatments. Deep learning can assist in various aspects of clinical trial design, including patient selection, dosage optimization, and establishing endpoints. Additionally, predictive modeling can identify potential challenges or roadblocks that may arise during the trial, enabling more informed decision-making and better allocation of resources.
During clinical trials, the volume and complexity of the data generated can be overwhelming. However, deep learning algorithms have the ability to handle such large datasets, ensuring accurate analysis and faster decision-making. Furthermore, these algorithms can identify patterns that might be missed by traditional statistical methods, uncovering valuable insights into treatment outcomes and drug responses.
In summary, deep learning has the potential to significantly impact how clinical studies are conducted in drug discovery. By integrating these advanced technologies, researchers can accelerate drug development, minimize costs, and ultimately contribute to delivering safer and more effective treatments for various health conditions.
Frequently Asked Questions
How do AI tools enhance drug discovery?
AI tools play a pivotal role in speeding up drug discovery and development by automating various processes and enhancing data analysis. Some ways that AI tools enhance drug discovery include advanced image analysis, prediction of molecular structure and function, and automated generation of molecules. By mining and interpreting vast datasets, AI-driven tools can identify potential drug candidates and optimize molecular properties with increased efficiency.
What are the benefits of using computer vision in drug discovery?
Computer vision, a subset of AI, can analyze and interpret images in drug discovery processes. By leveraging computer vision, researchers can quickly process and analyze large volumes of images such as cellular structures, protein conformations, and molecular simulations. These techniques can help identify drug targets, assess compound activity, and monitor complex biological processes, ultimately leading to better decision-making and increased productivity in drug discovery efforts.
What role does explainable AI play in drug discovery?
Explainable AI provides human-understandable insights into the underlying mechanisms and reasoning behind AI model predictions. In the context of drug discovery, explainable AI offers transparency and better understanding of decision processes, enabling researchers to explore and validate hypotheses and improve the models further. A prominent example is DrugEx, an open-source software package designed to provide insights into de novo drug design processes using a deep learning model.
Which machine learning models are popular in drug discovery?
Commonly used machine learning models in drug discovery include deep learning frameworks like convolutional neural networks (CNNs), recurrent neural networks (RNNs), and autoencoders. These models excel in handling complex data structures such as chemical compounds, bioactivity data, and molecular properties. Researchers also frequently use other machine learning algorithms like support vector machines (SVMs) and random forests for tasks like drug-target interaction prediction and ADMET property prediction.
How can deep learning improve structure-based drug discovery?
Structure-based drug discovery relies on accurate knowledge of the target protein’s 3D structure and its binding site. Deep learning models, such as CNNs and graph neural networks (GNNs), are effective in predicting protein 3D structures and capturing molecular interactions. By improving the understanding of protein conformation and ligand-receptor interactions, deep learning allows for more accurate virtual screening, docking simulations, and lead optimization, thus enhancing the overall structure-based drug discovery process.
What are the recent trends in ML-driven drug discovery?
Recent trends in ML-driven drug discovery include the use of transfer learning to leverage previously learned knowledge for new tasks, integration of multi-modal data sources to create more comprehensive models, and the adoption of GNNs to better capture molecular interactions. Some researchers are also focusing on the application of reinforcement learning techniques for optimizing molecule generation. Furthermore, the drug repurposing field has seen a surge of interest, with deep learning frameworks aiming to accelerate the process and reduce the risks associated with drug development.
2 thoughts on “Deep Learning for New Drug Treatment: Remarkable Breakthroughs”
Wonderful beat I wish to apprentice while you amend your web site how could i subscribe for a blog web site The account aided me a acceptable deal I had been a little bit acquainted of this your broadcast provided bright clear idea
Usually I do not read article on blogs however I would like to say that this writeup very compelled me to take a look at and do it Your writing style has been amazed me Thank you very nice article